Decentralized AI Systems: Benefits and Challenges
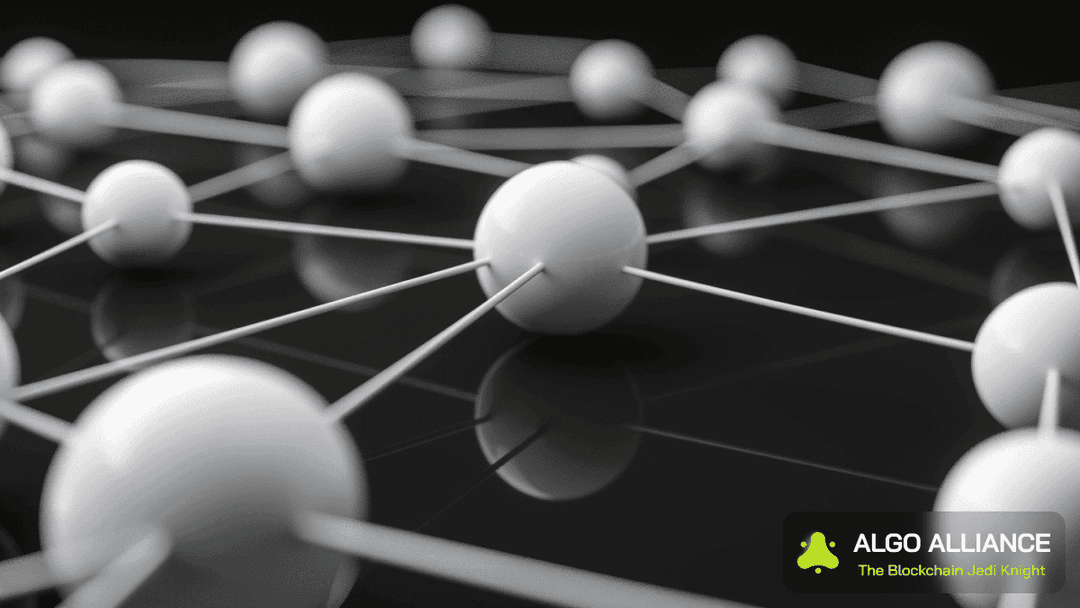

Erik Andersson
Published on 2023-08-29
|1 min read
Decentralized AI Systems: Benefits and Challenges
The rise of decentralized AI systems marks a significant shift in how artificial intelligence is developed, deployed, and managed. By leveraging blockchain technology, decentralized AI systems offer numerous benefits, from enhanced security and transparency to improved collaboration and innovation. However, this new paradigm also brings its own set of challenges that need to be addressed to realize its full potential. Here’s an exploration of the benefits and challenges associated with decentralized AI systems.
Benefits of Decentralized AI Systems
1. Enhanced Data Security and Privacy
One of the primary advantages of decentralized AI systems is the heightened security and privacy of data.
- Distributed Data Storage: Blockchain’s distributed ledger technology stores data across multiple nodes, reducing the risk of data breaches and single points of failure. This decentralization ensures that sensitive data is more secure compared to traditional centralized systems.
- Data Integrity: Blockchain creates immutable records of data transactions, preventing unauthorized alterations. This guarantees the integrity of the data used in AI training and decision-making processes, which is crucial for accurate and reliable outcomes.
2. Improved Transparency and Trust
Decentralized AI systems foster greater transparency and trust among stakeholders.
- Transparent Data Provenance: Blockchain provides a transparent and auditable trail of data provenance, allowing stakeholders to verify the origins and integrity of the data used in AI models. This transparency builds trust in the AI system’s outputs and decisions.
- Accountable AI Development: By recording every step of the AI development process on the blockchain, from data collection to model training, decentralized AI systems ensure that the development process is transparent and accountable. This can help mitigate biases and ensure ethical AI practices.
3. Enhanced Collaboration and Innovation
Decentralized AI systems promote collaboration and innovation by breaking down data silos and enabling secure data sharing.
- Federated Learning: Decentralized AI systems support federated learning, where AI models are trained across multiple decentralized devices without sharing raw data. This collaborative approach preserves data privacy and allows for more diverse and comprehensive training datasets.
- Incentivized Contributions: Blockchain-based AI platforms can use tokens and other incentives to reward data providers, developers, and users. This encourages active participation and contributions from a wide range of stakeholders, fostering innovation and enhancing the quality of AI models.
4. Resilience and Reliability
Decentralized AI systems are inherently more resilient and reliable than centralized counterparts.
- Censorship Resistance: The decentralized nature of blockchain makes AI systems more resilient against censorship and control by centralized authorities. This ensures continuous access to AI services and data, even in restrictive environments.
- Fault Tolerance: By distributing data and computation across multiple nodes, decentralized AI systems are less susceptible to failures. This fault tolerance enhances the reliability and availability of AI services, particularly in critical applications.
Challenges of Decentralized AI Systems
1. Scalability and Performance
One of the significant challenges of decentralized AI systems is achieving scalability and performance.
- Data Throughput: Blockchain networks can experience limitations in data throughput and latency, which can impact the performance of AI systems that require real-time data processing and high-speed computations.
- Computational Resources: Decentralized AI systems often require substantial computational resources to perform distributed training and inference. Ensuring that all nodes in the network have sufficient computational power can be challenging.
2. Complexity of Integration
Integrating blockchain and AI technologies to create decentralized AI systems involves significant complexity.
- Technical Expertise: Developing and managing decentralized AI systems requires expertise in both blockchain and AI technologies. This interdisciplinary knowledge can be challenging to acquire and maintain.
- Interoperability: Ensuring interoperability between different blockchain platforms and AI frameworks is crucial for seamless integration. However, achieving this interoperability can be technically complex and time-consuming.
3. Data Privacy and Compliance
While decentralized AI systems enhance data privacy, they also raise concerns related to data privacy and regulatory compliance.
- Data Anonymization: Ensuring that data remains anonymous while being used in decentralized AI systems can be challenging. Effective anonymization techniques are required to protect sensitive information without compromising the utility of the data.
- Regulatory Challenges: Navigating the regulatory landscape for data privacy and protection can be complex, especially when dealing with decentralized systems that operate across multiple jurisdictions. Ensuring compliance with regulations like GDPR and HIPAA is critical.
4. Governance and Accountability
Establishing effective governance and accountability mechanisms for decentralized AI systems is crucial but challenging.
- Decentralized Governance: Implementing decentralized governance models, such as Decentralized Autonomous Organizations (DAOs), requires careful design to ensure fair and transparent decision-making processes. Balancing decentralization with effective governance can be difficult.
- Ethical Considerations: Ensuring that decentralized AI systems adhere to ethical standards and do not perpetuate biases or discriminatory practices is essential. This requires ongoing monitoring, auditing, and updating of AI models and practices.
5. Resource Allocation and Incentives
Allocating resources and designing effective incentive mechanisms in decentralized AI systems pose unique challenges.
- Token Economics: Designing token economics that incentivize valuable contributions without creating unintended negative consequences is complex. Ensuring that tokens are fairly distributed and used effectively is crucial for the sustainability of decentralized AI systems.
- Resource Management: Efficiently managing computational resources and ensuring that they are allocated to the most critical tasks is essential. Decentralized systems need robust mechanisms to balance resource allocation and prevent wastage.
Decentralized AI systems, powered by blockchain technology, offer significant benefits in terms of security, transparency, collaboration, and resilience. However, they also present challenges related to scalability, integration, data privacy, governance, and resource management. Addressing these challenges requires interdisciplinary expertise, innovative solutions, and a commitment to ethical and transparent AI practices. As the field continues to evolve, the successful implementation of decentralized AI systems holds the potential to transform various industries and create a more secure, trustworthy, and equitable digital future