Blockchain and Machine Learning: Projects and Case Studies
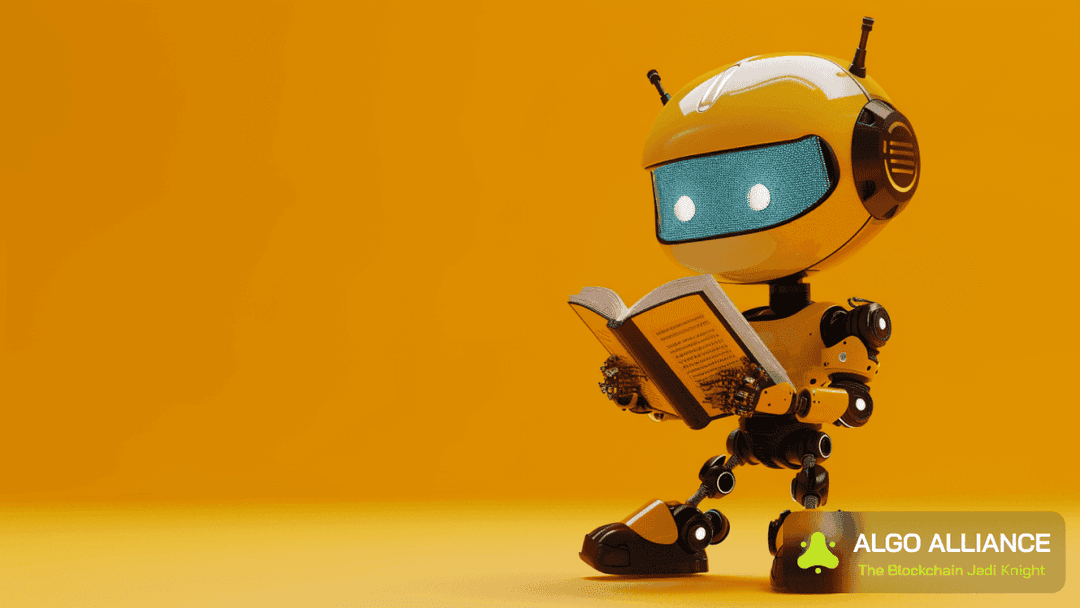

Erik Andersson
Published on 2023-09-26
|1 min read
Blockchain and Machine Learning: Projects and Case Studies
The convergence of blockchain and machine learning (ML) represents a groundbreaking synergy that offers innovative solutions across various industries. By combining the transparency and security of blockchain with the predictive and analytical power of machine learning, new possibilities emerge for enhancing data integrity, trust, and efficiency. This article explores notable projects and case studies that highlight the impact of integrating blockchain and ML.
Projects Utilizing Blockchain and Machine Learning
Several pioneering projects are at the forefront of integrating blockchain and ML, demonstrating the potential of these technologies when used in tandem.
- SingularityNET: This decentralized AI network allows developers to create, share, and monetize AI technologies securely. Leveraging blockchain, it ensures transparency and trust, enabling machine learning models to access a vast array of data sources while maintaining data integrity and privacy.
- Ocean Protocol: A decentralized data exchange protocol that combines blockchain with machine learning to unlock data for AI applications. By providing a secure and transparent marketplace, Ocean Protocol allows data owners to monetize their data while ensuring it is used ethically and securely by machine learning algorithms.
- Fetch.ai: A decentralized network that deploys autonomous agents capable ofperforming complex tasks, such as optimizing energy grids or managing supply chains. Fetch.ai leverages blockchain for secure data sharing and machine learning for predictive analytics and decision-making.
Case Studies Highlighting Blockchain and ML Integration
Real-world case studies provide insight into how blockchain and ML integration can solve complex problems and create value across various sectors.
1. Healthcare and Biomedical Research
- Medicalchain: This project uses blockchain to secure patient medical records, allowing authorized access and ensuring data integrity. Machine learning algorithms analyze the medical data to provide personalized treatment recommendations and predictive diagnostics, enhancing patient care and outcomes.
- Case Study: Project Enigma: At MIT, Enigma leverages blockchain to protect patient data while enabling secure computation of encrypted data using machine learning models. This approach allows researchers to train models on sensitive data without compromising patient privacy, leading to more accurate and effective healthcare solutions.
2. Finance and Fraud Detection
- Algorand: This blockchain platform employs machine learning to enhance security and efficiency in financial transactions. By analyzing transaction patterns, ML algorithms detect fraudulent activities and anomalies in real time, ensuring the integrity of financial operations.
- Case Study: Chainalysis: A blockchain analytics company that uses machine learning to monitor and analyze blockchain transactions. By identifying suspicious patterns and behaviors, Chainalysis helps financial institutions and regulators combat money laundering and fraud more effectively.
3. Supply Chain Management
- VeChain: A blockchain platform focused on enhancing supply chain transparency and efficiency. Machine learning algorithms analyze data collected from IoT devices across the supply chain to predict demand, optimize logistics, and ensure product authenticity.
- Case Study: IBM Food Trust: This blockchain-based supply chain solution integrates machine learning to analyze data from various stages of the food supply chain. By tracking and predicting potential issues, such as contamination or spoilage, IBM Food Trust improves food safety and reduces waste.
4. Energy Sector
- Grid+: A blockchain-based energy platform that uses machine learning to optimizeenergy distribution and consumption. By predicting energy demand and supply patterns, Grid+ enhances grid efficiency and reduces costs for consumers.
- Case Study: Power Ledger: This blockchain platform uses machine learning to manage and trade renewable energy. By analyzing energy production and consumption data, Power Ledger enables efficient peer-to-peer energy trading and ensures a balanced and sustainable energy grid.
Future Directions and Opportunities
The integration of blockchain and machine learning is still in its early stages, but the potential applications are vast and varied.
- Decentralized AI Marketplaces: Future projects may focus on creating decentralized marketplaces where AI and ML models can be securely shared and monetized using blockchain. These marketplaces will enable broader access to advanced technologies and promote collaborative innovation.
- Enhanced Data Privacy and Security: Combining blockchain’s immutable ledger with ML’s predictive power can create robust systems for managing and securing sensitive data. This will be particularly valuable in sectors such as healthcare, finance, and government, where data privacy is paramount.
- Smart Cities and IoT: Integrating blockchain and ML can optimize the operation of smart cities and IoT networks. From traffic management to energy distribution, these technologies can create more efficient, responsive, and sustainable urban environments.
The convergence of blockchain and machine learning represents a transformative shift in how data is managed, analyzed, and utilized across various industries. By exploring and implementing these innovative projects and case studies, organizations can unlock new levels of efficiency, security, and trust. As these technologies continue to evolve, their combined potential will drive significant advancements and open new frontiers in the digital landscape.